To view this email as a web page, click here. |
|
Happy New Year!
The recent Mascot Server release includes additional machine learning capabilities. Below, we explain what rescoring means and how it works.
In this month's highlighted publication, the authors characterise a sugar phosphate-processing bacterial microcompartment.
Protein inference is a critical and often misunderstood step – learn how Mascot does it.
|
|
|
|
|
 |
Mascot: The trusted reference standard for protein identification by mass spectrometry for 25 years
|
Get a quote
|
 |
|
How does rescoring with machine learning work?
|
|
A search against a protein sequence database is always a mixture of correct and incorrect peptide matches.
The Mascot ions score is designed so that the correct matches should mostly have high score and incorrect matches should mostly have low score, but it's not infallible.
Incorrect matches happen by chance for a number of reasons.
Maybe the correct sequence isn't in the database, or the spectrum has tall noise peaks that happen to match and score better against the wrong sequence.
The incorrect matches typically have systematic differences compared to correct matches, such as precursor mass error, charge state, number of missed cleavages, proportion of matched fragment ion intensity, and so on.
To make best use of this, Mascot includes Percolator, which uses semi-supervised machine learning to discover the factors that provide the strongest discrimination.
After choosing the best factors, Percolator condenses them into a single number, posterior error probability (PEP), which is used as the new match score (hence the term rescoring).
Most workflows benefit from enabling rescoring, as it often gives a substantial boost to sensitivity – even in routine database searches of human tryptic samples.
However, machine learning is not infallible either and can go wrong.
We believe it's important that you, the user, are able to decide whether to use machine learning, so you can enable or disable it as you choose.
A detailed discussion is in our blog.
|
|
|
 |
|
Featured publication using Mascot
Here we highlight a recent interesting and important publication that employs Mascot for protein identification, quantitation, or characterization. If you would like one of your papers highlighted here, please send us a PDF or a URL.
|
|
Characterization of a widespread sugar phosphate-processing bacterial microcompartment
Matthew E. Dwyer, Markus Sutter & Cheryl A. Kerfeld
Nature Communications Biology, volume 7, Article number: 1562 (2024)
Bacterial Microcompartments (BMCs) are formed by many prokaryotes and encapsulate segments of specialised metabolic pathways to enhance catalysis. The authors bioinformatically investigated a functionally uncharacterised BMC, the Sugar Phosphate Utilizing (SPU) BMC, loci of which are predicted to encode enzymes involved in sugar phosphate metabolism. They characterised seven SPU subtypes all containing an enzyme unique to SPU BMCs, a deoxyribose 5-phosphate aldolase (DERA), and another common SPU enzyme ribose 5-phosphate isomerase (RPI).
To functionally characterize these enzymes, the authors synthesized the coding regions with N-terminal HIS affinity tags for purification.
Because fusions of DERA and RPI exist in nature, the authors tested whether the two enzymes could form a complex.
Size exclusion chromatography showed one major peak around 131 kDa. SDS-PAGE analysis and Western blots of the peak fractions confirmed the presence of both RPI and DERA at about equal ratios.
This was confirmed by mass spectrometry analysis using Mascot Server, Mascot Distiller and Scaffold, and it is likely the first experimentally characterized interaction between DERA and RPI.
|
|
|
 |
|
Understanding protein inference
|
|
Protein inference is a critical and often misunderstood step when identifying proteins by shotgun LC-MS/MS.
Because the database search identifies only peptides, the software has to reconstruct the most likely protein content of the sample from the identified peptides.
Mascot implements a novel algorithm that uses information from both unique and shared peptides to build a complete picture.
First, proteins are clustered into "families" based on shared peptide matches.
Then, protein hits with unique evidence (unique peptides) are promoted into top-level family members.
All other proteins, which lack unique evidence, are relegated into subset status and not displayed by default.
In short, every top-level protein hit reported by Mascot is supported by unique MS/MS evidence, which you can confirm by viewing the peptide fragmentation and matched ions.
The Mascot online help includes a full description of the algorithm, including examples.
|
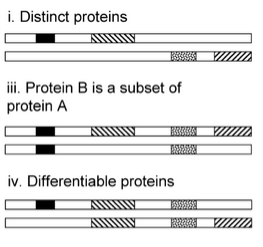 |
|
 |
|
About Matrix Science
Matrix Science is a provider of bioinformatics tools to proteomics researchers and scientists, enabling the rapid, confident identification and quantitation of proteins. Mascot continues to be cited by over 2000 publications every year. Our software products fully support data from mass spectrometry instruments made by Agilent, Bruker, Sciex, Shimadzu, Thermo Scientific, and Waters.
Get a quote
|
|
You can also contact us or one of our marketing partners for more information on how you can power your proteomics with Mascot.
|
|
|
|
|